TPJ Editor choice - June 2024: Use the needle in the haystack: Spike-ins as a normalization for RNAseq
Use the needle in the haystack: Spike-ins as a normalization for RNAseq
In the mid-2000s, high throughput sequencing replaced the use of micro-arrays, and has been used since then to study gene expression, alternative splicing and cis-regulatory elements. Sample preparation affects the total number of transcripts in the sample, and thereby the sequencing coverage. Commonly used tools for analysing differential expression normalize for read depth based on the assumption that expression of most genes does not change between samples, i.e. that the overall RNA abundance between samples is the same. However, many experimental conditions can affect the level of transcription or RNA stability, and therefore total RNA abundance.
For a more reliable differential expression analysis, Laosuntisuk et al. explored the use of RNA spike-ins as a way of normalizing the samples. RNA spike-ins are synthetic control RNAs that are added to the isolated RNA of each sample, and serve as a scale for the subsequent differential expression analysis (Figure 1). They are commonly used for RNAseq experiments in yeast, animal and human samples, but less frequently in plants.
First, the authors used a synthetic dataset to demonstrate the effect of RNA spike-ins. They created a dataset of samples in two conditions, in which the samples of the second condition comprised more reads per sample. The classical ‘Median of Ratio’ normalization method calculates the median of the read count ratio in one sample to a geometric mean across all samples. By this method, transcript abundance was scaled between the two conditions to the same level, and therefore did not represent the difference in total transcript abundance under the second condition. Using RNA spike-ins, however, reflected the increased transcript abundance in the samples under the second condition, suggesting that the spike-in control removed the technical variations but maintained global differences in transcript abundance.
Then, the authors tested the spike-in normalization on a dataset of RNA from sorghum leaves, sampled under control and chilling stress conditions at both morning and evening time points. The samples collected at the evening had a higher average read count than the ones collected in the morning. As in the artificial dataset, the classical normalization method removed the variation between replicates and aligned all samples to the same level, while spike-in normalization removed the variation between replicates but kept the variation between samples. Additionally, the authors detected upregulated genes in the evening sample with the spike-in normalization that were not detected by the traditional method. This suggests that the reduced variation between samples of a specific condition, and the increase of the average expression in all evening samples influenced the statistical significance of their difference, the total change in expression level, or both. Gene Ontology enrichment analysis showed that evening-specific functions and cellular processes may be under-represented or missed using traditional DEG analysis. Furthermore, the spike-in normalization identified more chilling down-regulated genes in the morning, but more chilling up-regulated genes in the evening, while the traditional method detected similar numbers both morning and evening.
In summary, this suggests that the use of RNA spike-ins improves DEG identification when the overall gene expression is altered between different samples. This might be particularly valuable for single cell RNAseq experiments, in which transcript abundance is highly variable between samples and not all transcripts can be captured and sequenced due to cell-specific inefficiencies in reverse transcription.
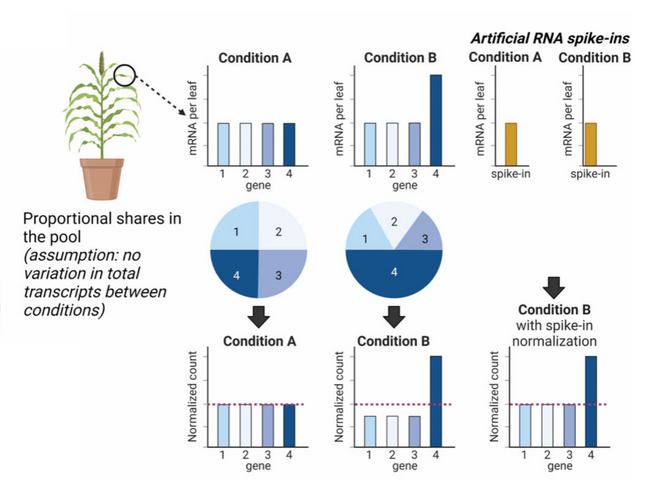
Figure 1: RNA spike-ins as a normalization method for RNAseq.
If one experimental condition (Condition B) alters the expression of a specific group of genes, and the dataset is normalized based on transcript abundance, the expression of the other genes is underrepresented under that condition (left); RNA spike-ins maintain the proportion between the gene groups (right).